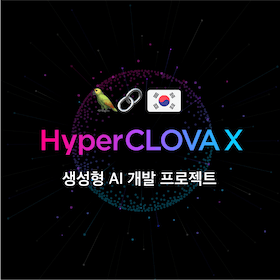
종료
100만원 네이버페이 + 150만원 크레딧
랭체인 코리아
팔로워81명
주최대회0개
Competition
전체보기종료
100만원 네이버페이 + 150만원 크레딧
종료
100만원 네이버페이 + 150만원 크레딧
종료
종료
종료
Learning
전체보기종료
종료
종료
종료
종료
종료
종료
Training
전체보기진행중
진행중
진행중
진행중
진행중
진행중
진행중
Chat
전체보기Forum
전체보기Hello, everyone! This is Taeyoung Kim from LangChain Korea. 😄 Our LangChain Korea 2025Q1 Meetup on the theme of "Agentic AI" was a great success, with a total of 164 attendees (143 participants, 11 speakers, and 10 organizers & staff)! 🎉 This event focused on Agentic AI, a rising trend in the AI industry, covering: Single-agent systems, Multi-agent architectures, LangGraph, Various frameworks and real-world applications. A total of 11 expert speakers delivered sessions packed with practical insights and case studies that can be directly applied to real-world projects. 💡 Now, let's take a look at some highlights from the event! 📸🎬 Behind the Scenes: Getting Ready!📍 The meetup took place on the 13th floor of Microsoft Korea! The spacious venue, large screens, and well-arranged tables made it an ideal setting for presentations. ❄️ Despite the snowy weather across the country, many attendees traveled from afar to join us—thank you for your dedication! 🙏🎥 Special Message from LangChain Team's Jess!Jess from LangChain Team sent a special message celebrating our event! 🎊 She also shared the latest updates on the Interrupt(https://interrupt.langchain.com/). ✨ We truly appreciate the warm support from afar! 💙 💙 Consistent Support from Microsoft Korea & Space-S🎙️ Director Soyoung Lee from Microsoft Korea delivered a welcome speech! Fun fact: reserving an entire floor for an event requires months of preparation, and Microsoft's staff provided incredible support throughout the event! 👀 A huge thank you to Microsoft Korea for sponsoring our venue! 🙌 🚀 CEO Choongil Lee also shared inspiring words about building "Warm AI" through innovation. Thank you for your continued support!🏆 Exciting News! 🎉🥳 TeddyNote (Kyungrok Lee) has been officially appointed as a LangChain Ambassador! A huge congratulations! 👏👏👏📅 Session Schedule🔥 A non-stop, 6-hour knowledge-packed event from 3:00 PM to 9:00 PM! The dedication from our participants was truly inspiring. 💪 For the next meetup, we plan to include networking opportunities and a dinner break! 😆⏰ Session ListTimeSessionSpeaker15:00 - 15:25Building Multi-Agent Collaborative Networks with LangGraphKyungrok Lee (BrainCrew)15:30 - 15:55Building Agentic AI Systems with AssistWorksTaeyoung Kim (AI Factory)16:00 - 16:25Building Systems with PydanticAIJungjoon Heo (Engineer)16:30 - 16:55The Future of Search in the Agentic AI EraSeungyoon Baek (Engineer)17:00 - 17:25AI Collaboration Systems Using OpenAI SwarmHogan Seo (Korea Atomic Energy Research Institute)17:30 - 17:55Building an Agentic Search System: A Focus on Autonomy LevelsHoon Heo (Engineer)18:30 - 18:55The Future of Productivity with Agentic AIJiwon Seol (Engineer)19:00 - 19:25System Development with Microsoft Magentic-OneSeongjae Yoon (AI Consultant)19:30 - 19:55Building Agentic AI Systems with CrewAIJinhyung Lee (Engineer)20:00 - 20:25Building AI Systems with Copilot StudioHyunah Yoo (IT Content Creator)20:30 - 20:55Developing Multi-Agent Systems for Enterprises with AutoGenWonseok Jung (AI Researcher)15:00 ~ 15:25 Building Multi-Agent Collaborative Networks with LangGraph, Kyungrok Lee (BrainCrew)15:30 ~ 15:55 Building Agentic AI Systems with AssiWorks, Taeyoung Kim (AI Factory)16:00 ~ 16:25 Building Systems with PydanticAI, Jungjoon Heo (Engineer)16:30 ~ 16:55 The Future of Search in the Agentic AI Era, Seungyoon Baek (Engineer)17:00 ~ 17:25 AI Collaboration Systems Using OpenAI Swarm, Hogan Seo (Korea Atomic Energy Research Institute)17:30 ~ 17:55 Building an Agentic Search System: A Focus on Autonomy Levels, Hoon Heo (Engineer)18:30 ~ 18:55 The Future of Productivity with Agentic AI, Jiwon Seol (Engineer)19:00 ~ 19:25 System Development with Microsoft Magentic-One, Seongjae Yoon (AI Consultant)19:30 ~ 19:55 Building Agentic AI Systems with CrewAI, Jinhyung Lee (Engineer)20:00 ~ 20:25 Building AI Systems with Copilot Studio, Hyunah Yoo (IT Content Creator)20:30 ~ 20:55 Developing Multi-Agent Systems for Enterprises with AutoGen, Wonseok Jung (AI Researcher)🔥 The Unstoppable Passion of Our Participants!📚 From 3:00 PM to 9:00 PM, without breaks, fully focused on learning! Participants actively engaged in discussions, knowledge sharing, and deep dives into Agentic AI. For the next event, we will add networking and dinner breaks to make it even better! 😆 📸 Here’s a snapshot of our final “chain pose” of LangChain group photo! 📷💪 LangChain Korea Organizer Team🎤 A huge shoutout to the LangChain Korea organizers who planned, prepared, and managed this amazing event! 👏 (From left to right: Taeyoung Kim, Seoyeon Jang, Jiseok Lee, Hyunah Yoo, Mijeong Jeon) Thank you for making this possible! 💙 Please continue to support and engage with the LangChain Korea Community!🚀 Stay Tuned for the Next Meetup! We’ll be back with even more valuable content and networking opportunities! Thank you all, and see you next time! 🙌💙📢 Presentation Materials & Recorded Sessions 🎥📑We will soon share presentation slides and recorded videos with attendees! ⏳📩 Stay tuned for updates, and get ready to revisit the key insights from the event! 🚀✨
안녕하세요! 랭체인코리아의 김태영입니다. 😄 지난 랭체인코리아 2025Q1 밋업이 "에이전틱 AI"를 주제로 총 164명(참가자 143명, 연사 11명, 오거나이저 및 스태프 10명)이 모여 성황리에 마무리되었습니다! 🎉 이번 밋업에서는 최근 AI 업계에서 주목받고 있는 에이전틱 AI를 중심으로 진행되었으며, 단일 에이전트부터 멀티 에이전트 시스템과 다양한 프레임워크까지 폭넓게 다뤘습니다. 총 11명의 전문가가 각기 다른 세션에서 실무에서 바로 적용할 수 있는 기술과 다양한 사례를 공유해 주셨는데요.💡 함께 현장 스케치를 살펴볼까요? 📸🎬 준비하는 현장부터 시작!📍 이번 밋업 장소는 한국마이크로소프트 13층이었습니다! 넓고 쾌적한 공간 덕분에 긴 스크린과 넓은 책상이 마련되어 있어 발표 슬라이드를 보기에 최적이었어요. ❄️ 전국 곳곳에 눈이 내리는 날씨에도 멀리 지방에서 와주신 참가자분들께 진심으로 감사드립니다! 🙏🎥 LangChain Team의 Jess님 축전!LangChain Team의 Jess님께서 본 행사를 위해 축전을 보내주셨습니다! 🎊 또한, 최신 소식인 Interrupt 행사(https://interrupt.langchain.com/) 도 함께 소개해 주셨어요.✨ 이렇게 멀리서도 따뜻한 인사를 전해주셔서 감사드립니다! 💙 변함없는 후원: 한국마이크로소프트 & 대덕특구 Space-S🎙️ 마이크로소프트 이소영 이사님께서 환영 인사를 전해주셨습니다! 사실 한 층 전체를 예약하려면 몇 달 전부터 준비해야 하고, 행사 당일에도 마이크로소프트 직원분들의 서포트가 필요하다는 사실, 알고 계셨나요? 👀 매번 장소를 후원해 주시는 마이크로소프트에 다시 한번 감사드립니다. 🙌🚀 이충일 대표님께서 "따뜻한 AI"를 만들기 위한 메시지도 전해주셨습니다. 감사합니다!🏆 축하할 소식! 🎉🥳 테디노트(이경록)님께서 랭체인 공식 엠배서더로 선정되셨습니다! 이 자리를 빌려 진심으로 축하드립니다! 👏👏👏📅 세션 일정🔥 오후 3시부터 밤 9시까지, 6시간 논스톱 세션! 참가자분들의 열정이 정말 대단했는데요! 💪 다음 밋업에서는 네트워킹 및 저녁 시간도 마련해 보겠습니다. 😆⏰ Session List시간세션발표자15:00 ~ 15:25LangGraph를 활용한 멀티에이전트 협업 네트워크 시스템 구축이경록 (브레인크루)15:30 ~ 15:55어시웍스를 활용한 에이전틱 AI 시스템 구축김태영 (인공지능팩토리)16:00 ~ 16:25PydanticAI를 활용한 시스템 구축 방법허정준 (Engineer)16:30 ~ 16:55에이전틱 AI가 가져올 검색의 미래백승윤 (Engineer)17:00 ~ 17:25OpenAI Swarm을 활용한 AI 협업 시스템 구축서호건 (한국원자력연구원)17:30 ~ 17:55에이전틱 서치 시스템 구축 - 높아진 Autonomy Level을 중심으로허훈 (Engineer)18:30 ~ 18:55에이전틱 AI와 생산성의 미래설지원 (Engineer)19:00 ~ 19:25Microsoft Magentic-One을 활용한 시스템 구축윤성재 (AI 컨설턴트)19:30 ~ 19:55CrewAI를 활용한 에이전틱 AI 시스템 구축이진형 (Engineer)20:00 ~ 20:25Copilot Studio를 활용한 AI 시스템 구축유현아 (IT Content Creator)20:30 ~ 20:55AutoGen을 활용한 기업을 위한 멀티에이전트 구축정원석 (AI 연구원)15:00 ~ 15:25 LangGraph를 활용한 멀티에이전트 협업 네트워크 시스템 구축 (이경록, 브레인크루)15:30 ~ 15:55 어시웍스를 활용한 에이전틱AI 시스템 구축 (김태영, 인공지능팩토리)16:00 ~ 16:25 PydanticAI를 활용한 시스템 구축 방법 (허정준, Engineer)16:30 ~ 16:55 에이전틱 AI가 가져올 검색의 미래 (백승윤, Engineer)17:00 ~ 17:25 OpenAI Swarm를 활용한 AI 협업 시스템 구축 (서호건, 한국원자력연구원)17:30 ~ 17:55 에이전틱 서치 시스템 구축 | 높아진 Autonomy Level을 중심으로 (허훈, Engineer)18:30 ~ 18:55 에이전틱 AI와 생산성의 미래 (설지원, Engineer)19:00 ~ 19:25 Microsoft Magentic-One를 활용한 시스템 구축 (윤성재, AI 컨설턴트)19:30 ~ 19:55 CrewAI를 활용한 에이전틱AI 시스템 구축 (이진형 | Engineer)20:00 ~ 20:25 Copilot Studio를 활용한 AI 시스템 구축 (유현아, IT Content Creator)20:30 ~ 20:55 AutoGen를 활용한 기업을 위한 멀티에이전트 구축 (정원석, AI 연구원)🔥 참가자들의 열정, 그 자체!📚 오후 3시부터 밤 9시까지, 쉬는 시간 없이 풀 집중 모드! 모두가 열정적으로 지식을 공유하고, 배우고, 토론한 정말 뜻깊은 시간이었습니다! 다음에는 네트워킹 시간과 저녁 시간도 추가하겠습니다.😆 📸 마지막까지 남아 체인을 상징하는 포즈로 단체 사진도 찍었습니다! 📷💪 랭체인코리아 오거나이저 팀🎤 본 행사를 사전에 기획, 준비, 운영한 랭체인코리아 오거나이저 팀(왼쪽부터 김태영, 장서연, 이지석, 유현아, 전미정)입니다! 👏 앞으로도 랭체인코리아 커뮤니티에 많은 관심과 사랑 부탁드립니다!📢 다음 밋업도 기대해 주세요! 🚀더욱 유익한 콘텐츠와 네트워킹 기회로 다시 찾아뵙겠습니다! 감사합니다! 🙌💙📢 발표자료 및 녹화영상 안내 🎥📑조만간 참가자분들에게 발표자료 및 녹화영상에 대한 안내가 나갈 예정이니 기다려주세요! ⏳📩 잊지 않고 공유해 드릴 테니 많은 기대 부탁드립니다! 🚀✨
OpenAI의 ChatCompletion API에서 Function Calling(함수 호출) 기능을 통해 모델이 스스로 적절한 함수를 선택하고, 필요한 인자를 구성하여 호출할 수 있게 되었습니다. 기존에는 모델의 답변을 파싱하여 사용자가 직접 “필요한 정보를 추출”하곤 했지만, 이제는 모델이 명시적으로 “특정 함수”를 호출하고, 그 결과를 받아 다음 문맥에 활용할 수 있습니다.이 글에서는 a부터 z까지 총 26개의 함수가 서로 의존성을 갖는 예시를 통해, Function Calling을 어떻게 구성할 수 있는지 살펴보겠습니다. 각 함수는 이전 함수가 만들어낸 “토큰”을 입력받아 새로운 “토큰”을 생성하고, 마지막에 a_func가 최종 결과를 만들어내는 구조입니다.1. 시나리오: a_func를 호출하기 위해 z_func부터 차례대로 호출예시 상황은 다음과 같습니다.z_func: 의존성이 전혀 없는 함수. 내부적으로 "z"라는 토큰을 만듦y_func: z_token을 받아서 "y" + z_token을 리턴x_func: y_token → "x" + y_token…b_func: c_token → "b" + c_tokena_func: b_token → "a" + b_token (최종 결과)사용자는 “A 함수를 호출해”라고만 말해도, 모델은 z_func → y_func → x_func → ... → b_func → a_func 순으로 호출해야 한다는 사실을 인식하고, 중간 단계 함수들을 모두 호출할 수 있습니다.2. 전체 예시 코드아래 코드에서는:z_func ~ a_func: 파이썬 상의 실제 함수들(문자열을 이어붙이는 단순 예시)tools: OpenAI에 노출할 함수의 정의(각 함수 이름, 설명, 파라미터 스키마)toolkits: 함수 이름과 실제 파이썬 함수의 매핑openai_llm(...): ChatCompletion API를 호출하고, 모델이 함수 호출을 요청하면 실제로 파이썬 함수를 실행하여 결과를 다시 모델에게 전달(while 루프로 재시도)코드 전문<code class="language-python"># -*- coding: utf-8 -*- """ function_calling_demo.py OpenAI의 Function Calling 기능을 활용해, a_func부터 z_func까지 26개의 함수가 서로 의존성을 가지도록 구성한 예시 코드. '도구(tools)' 정의를 통해 모델이 필요할 때 각 함수를 호출하고, 함수 결과를 다시 모델에 전달하는 과정을 반복하여 최종 값을 얻는다. """ from openai import OpenAI import requests import json import yfinance as yf ######################################## # 1. OpenAI 클라이언트 초기화 ######################################## client = OpenAI( api_key="sk-p..." ) ######################################## # 2. a~z 함수 정의 # - z_func: 의존성 없는 가장 기초 # - y_func: z_token 필요 # - ... # - a_func: b_token 필요(최종) # # 실제 로직(토큰 생성 등)은 단순히 문자열을 이어붙이는 것으로 예시 구성 ######################################## def z_func(): """z_func: 의존성 없음, 결과로 'z'를 리턴""" return "z" def y_func(z_token): """y_func: z_token -> 'y' + z_token""" return "y" + z_token def x_func(y_token): """x_func: y_token -> 'x' + y_token""" return "x" + y_token def w_func(x_token): """w_func: x_token -> 'w' + x_token""" return "w" + x_token def v_func(w_token): """v_func: w_token -> 'v' + w_token""" return "v" + w_token def u_func(v_token): """u_func: v_token -> 'u' + v_token""" return "u" + v_token def t_func(u_token): """t_func: u_token -> 't' + u_token""" return "t" + u_token def s_func(t_token): """s_func: t_token -> 's' + t_token""" return "s" + t_token def r_func(s_token): """r_func: s_token -> 'r' + s_token""" return "r" + s_token def q_func(r_token): """q_func: r_token -> 'q' + r_token""" return "q" + r_token def p_func(q_token): """p_func: q_token -> 'p' + q_token""" return "p" + q_token def o_func(p_token): """o_func: p_token -> 'o' + p_token""" return "o" + p_token def n_func(o_token): """n_func: o_token -> 'n' + o_token""" return "n" + o_token def m_func(n_token): """m_func: n_token -> 'm' + n_token""" return "m" + n_token def l_func(m_token): """l_func: m_token -> 'l' + m_token""" return "l" + m_token def k_func(l_token): """k_func: l_token -> 'k' + l_token""" return "k" + l_token def j_func(k_token): """j_func: k_token -> 'j' + k_token""" return "j" + k_token def i_func(j_token): """i_func: j_token -> 'i' + j_token""" return "i" + j_token def h_func(i_token): """h_func: i_token -> 'h' + i_token""" return "h" + i_token def g_func(h_token): """g_func: h_token -> 'g' + h_token""" return "g" + h_token def f_func(g_token): """f_func: g_token -> 'f' + g_token""" return "f" + g_token def e_func(f_token): """e_func: f_token -> 'e' + f_token""" return "e" + f_token def d_func(e_token): """d_func: e_token -> 'd' + e_token""" return "d" + e_token def c_func(d_token): """c_func: d_token -> 'c' + d_token""" return "c" + d_token def b_func(c_token): """b_func: c_token -> 'b' + c_token""" return "b" + c_token def a_func(b_token): """a_func: b_token -> 'a' + b_token (최종)""" return "a" + b_token ############################################## # 3. tools 정의 (Function Calling용 스키마) # - a~z 모든 함수를 등록하고, 각 함수에 필요한 인자와 설명을 JSON Schema로 표시 ############################################## tools = [ { "type": "function", "function": { "name": "z_func", "description": ( "Z 함수: 의존성이 없는 가장 기초 함수. 결과값으로 z_token을 " "생성한다고 가정. 다른 많은 함수들이 z_token을 필요로 할 수 있음." ), "parameters": { "type": "object", "properties": { # z_func는 인자가 없음 }, "required": [] }, }, }, { "type": "function", "function": { "name": "y_func", "description": ( "Y 함수: z_token이 필요. z_func의 결과로부터 z_token을 받아서 " "y_token을 생성한다고 가정." ), "parameters": { "type": "object", "properties": { "z_token": { "type": "string", "description": "z_func 호출 결과로 얻은 토큰" } }, "required": ["z_token"] }, }, }, { "type": "function", "function": { "name": "x_func", "description": ( "X 함수: y_token이 필요. y_func의 결과로부터 y_token을 받아서 " "x_token을 생성한다고 가정." ), "parameters": { "type": "object", "properties": { "y_token": { "type": "string", "description": "y_func 호출 결과로 얻은 토큰" } }, "required": ["y_token"] }, }, }, { "type": "function", "function": { "name": "w_func", "description": "W 함수: x_token 필요, 결과로 w_token을 만든다고 가정.", "parameters": { "type": "object", "properties": { "x_token": { "type": "string", "description": "x_func 호출 결과로 얻은 토큰" } }, "required": ["x_token"] }, }, }, { "type": "function", "function": { "name": "v_func", "description": "V 함수: w_token 필요, 결과로 v_token을 만든다고 가정.", "parameters": { "type": "object", "properties": { "w_token": { "type": "string", "description": "w_func 호출 결과로 얻은 토큰" } }, "required": ["w_token"] }, }, }, { "type": "function", "function": { "name": "u_func", "description": "U 함수: v_token 필요, 결과로 u_token을 만든다고 가정.", "parameters": { "type": "object", "properties": { "v_token": { "type": "string", "description": "v_func 호출 결과로 얻은 토큰" } }, "required": ["v_token"] }, }, }, { "type": "function", "function": { "name": "t_func", "description": "T 함수: u_token 필요, 결과로 t_token을 만든다고 가정.", "parameters": { "type": "object", "properties": { "u_token": { "type": "string", "description": "u_func 호출 결과로 얻은 토큰" } }, "required": ["u_token"] }, }, }, { "type": "function", "function": { "name": "s_func", "description": "S 함수: t_token 필요, 결과로 s_token을 만든다고 가정.", "parameters": { "type": "object", "properties": { "t_token": { "type": "string", "description": "t_func 호출 결과로 얻은 토큰" } }, "required": ["t_token"] }, }, }, { "type": "function", "function": { "name": "r_func", "description": "R 함수: s_token 필요, 결과로 r_token을 만든다고 가정.", "parameters": { "type": "object", "properties": { "s_token": { "type": "string", "description": "s_func 호출 결과로 얻은 토큰" } }, "required": ["s_token"] }, }, }, { "type": "function", "function": { "name": "q_func", "description": "Q 함수: r_token 필요, 결과로 q_token을 만든다고 가정.", "parameters": { "type": "object", "properties": { "r_token": { "type": "string", "description": "r_func 호출 결과로 얻은 토큰" } }, "required": ["r_token"] }, }, }, { "type": "function", "function": { "name": "p_func", "description": "P 함수: q_token 필요, 결과로 p_token을 만든다고 가정.", "parameters": { "type": "object", "properties": { "q_token": { "type": "string", "description": "q_func 호출 결과로 얻은 토큰" } }, "required": ["q_token"] }, }, }, { "type": "function", "function": { "name": "o_func", "description": "O 함수: p_token 필요, 결과로 o_token을 만든다고 가정.", "parameters": { "type": "object", "properties": { "p_token": { "type": "string", "description": "p_func 호출 결과로 얻은 토큰" } }, "required": ["p_token"] }, }, }, { "type": "function", "function": { "name": "n_func", "description": "N 함수: o_token 필요, 결과로 n_token을 만든다고 가정.", "parameters": { "type": "object", "properties": { "o_token": { "type": "string", "description": "o_func 호출 결과로 얻은 토큰" } }, "required": ["o_token"] }, }, }, { "type": "function", "function": { "name": "m_func", "description": "M 함수: n_token 필요, 결과로 m_token을 만든다고 가정.", "parameters": { "type": "object", "properties": { "n_token": { "type": "string", "description": "n_func 호출 결과로 얻은 토큰" } }, "required": ["n_token"] }, }, }, { "type": "function", "function": { "name": "l_func", "description": "L 함수: m_token 필요, 결과로 l_token을 만든다고 가정.", "parameters": { "type": "object", "properties": { "m_token": { "type": "string", "description": "m_func 호출 결과로 얻은 토큰" } }, "required": ["m_token"] }, }, }, { "type": "function", "function": { "name": "k_func", "description": "K 함수: l_token 필요, 결과로 k_token을 만든다고 가정.", "parameters": { "type": "object", "properties": { "l_token": { "type": "string", "description": "l_func 호출 결과로 얻은 토큰" } }, "required": ["l_token"] }, }, }, { "type": "function", "function": { "name": "j_func", "description": "J 함수: k_token 필요, 결과로 j_token을 만든다고 가정.", "parameters": { "type": "object", "properties": { "k_token": { "type": "string", "description": "k_func 호출 결과로 얻은 토큰" } }, "required": ["k_token"] }, }, }, { "type": "function", "function": { "name": "i_func", "description": "I 함수: j_token 필요, 결과로 i_token을 만든다고 가정.", "parameters": { "type": "object", "properties": { "j_token": { "type": "string", "description": "j_func 호출 결과로 얻은 토큰" } }, "required": ["j_token"] }, }, }, { "type": "function", "function": { "name": "h_func", "description": "H 함수: i_token 필요, 결과로 h_token을 만든다고 가정.", "parameters": { "type": "object", "properties": { "i_token": { "type": "string", "description": "i_func 호출 결과로 얻은 토큰" } }, "required": ["i_token"] }, }, }, { "type": "function", "function": { "name": "g_func", "description": "G 함수: h_token 필요, 결과로 g_token을 만든다고 가정.", "parameters": { "type": "object", "properties": { "h_token": { "type": "string", "description": "h_func 호출 결과로 얻은 토큰" } }, "required": ["h_token"] }, }, }, { "type": "function", "function": { "name": "f_func", "description": "F 함수: g_token 필요, 결과로 f_token을 만든다고 가정.", "parameters": { "type": "object", "properties": { "g_token": { "type": "string", "description": "g_func 호출 결과로 얻은 토큰" } }, "required": ["g_token"] }, }, }, { "type": "function", "function": { "name": "e_func", "description": "E 함수: f_token 필요, 결과로 e_token을 만든다고 가정.", "parameters": { "type": "object", "properties": { "f_token": { "type": "string", "description": "f_func 호출 결과로 얻은 토큰" } }, "required": ["f_token"] }, }, }, { "type": "function", "function": { "name": "d_func", "description": "D 함수: e_token 필요, 결과로 d_token을 만든다고 가정.", "parameters": { "type": "object", "properties": { "e_token": { "type": "string", "description": "e_func 호출 결과로 얻은 토큰" } }, "required": ["e_token"] }, }, }, { "type": "function", "function": { "name": "c_func", "description": "C 함수: d_token 필요, 결과로 c_token을 만든다고 가정.", "parameters": { "type": "object", "properties": { "d_token": { "type": "string", "description": "d_func 호출 결과로 얻은 토큰" } }, "required": ["d_token"] }, }, }, { "type": "function", "function": { "name": "b_func", "description": "B 함수: c_token 필요, 결과로 b_token을 만든다고 가정.", "parameters": { "type": "object", "properties": { "c_token": { "type": "string", "description": "c_func 호출 결과로 얻은 토큰" } }, "required": ["c_token"] }, }, }, { "type": "function", "function": { "name": "a_func", "description": ( "A 함수: b_token이 필요. b_func 호출 결과로 b_token을 받고, " "최종 결과를 생성한다고 가정." ), "parameters": { "type": "object", "properties": { "b_token": { "type": "string", "description": "b_func 호출 결과로 얻은 토큰" } }, "required": ["b_token"] }, }, } ] ############################################## # 4. 실제 Python 함수(코드)와 tool name 매핑 ############################################## toolkits = { "z_func": z_func, "y_func": y_func, "x_func": x_func, "w_func": w_func, "v_func": v_func, "u_func": u_func, "t_func": t_func, "s_func": s_func, "r_func": r_func, "q_func": q_func, "p_func": p_func, "o_func": o_func, "n_func": n_func, "m_func": m_func, "l_func": l_func, "k_func": k_func, "j_func": j_func, "i_func": i_func, "h_func": h_func, "g_func": g_func, "f_func": f_func, "e_func": e_func, "d_func": d_func, "c_func": c_func, "b_func": b_func, "a_func": a_func, } ######################################## # 5. 시스템 메시지 # - 모델에게 "알아서 필요한 함수는 호출하라" 고 지시 ######################################## system_prompt = """ You are a helpful assistant. Write in Korean. You have access to the following tools. If the user asks for something that requires calling a function, call it with the correct arguments. Otherwise, respond directly in natural language. """ ######################################## # 6. 대화(챗) + 함수 호출 로직 ######################################## def openai_llm(user_input, chat_history): """ - chat_history에 대화 내용(system, user, assistant, tool 등)을 순차적으로 기록 - 모델에게 질의 후, 만약 tool_calls가 있으면 해당 함수를 실제로 호출하고, 그 결과를 tool 메시지로 추가 - 다시 모델에게 재요청을 반복하여, 함수 호출이 더 이상 없을 때 최종 답변을 반환 """ # 최초 대화에 system 메시지를 넣음 if len(chat_history) == 0: chat_history.append({"role": "system", "content": system_prompt}) # 사용자 메시지를 추가 chat_history.append({"role": "user", "content": user_input}) # 함수 호출이 없을 때까지 반복 while True: # 1) OpenAI ChatCompletion 요청 completion = client.chat.completions.create( model="gpt-4o", # 예시 모델 messages=chat_history, tools=tools ) # 모델이 생성한 assistant 메시지를 획득 assistant_msg = completion.choices[0].message # assistant_msg를 대화 기록에 추가 chat_history.append(assistant_msg) # 2) 모델이 함수 호출을 했는지 (tool_calls) 확인 if not hasattr(assistant_msg, "tool_calls") or not assistant_msg.tool_calls: # 함수 호출이 없으면 일반 답변으로 간주, while 종료 break # 3) 함수 호출이 있을 경우 -> 실제 파이썬 함수를 호출 for tool_call in assistant_msg.tool_calls: fn_name = tool_call.function.name fn_args_str = tool_call.function.arguments # 함수 인자를 JSON 디코딩 fn_args = json.loads(fn_args_str) if fn_args_str else {} print(fn_name, fn_args_str) # 디버그 출력 (어떤 함수가 어떻게 호출되는지) # 실제 함수 실행 result = toolkits[fn_name](**fn_args) # 실행 결과를 role="tool" 메시지로 추가 tool_message = { "role": "tool", "tool_call_id": tool_call.id, "content": result } chat_history.append(tool_message) # 4) tool 메시지를 추가한 뒤, while 루프를 반복하여 # 모델이 다시 한 번(또는 여러 번) 함수 호출할지 확인 # 없으면 break # 함수 호출이 없을 때(while 탈출 시), 최종적으로 assistant_msg.content 리턴 return assistant_msg.content ######################################## # 7. 실행 예시 ######################################## if __name__ == "__main__": # 첫 테스트: "A 함수를 호출해"라고 요청해보기 # 모델이 z부터 b까지 차례로 호출 후 최종적으로 a_func를 호출하는지 확인 answer = openai_llm("A 함수를 호출해", []) print("A I >", answer) # 대화 루프 예시 # - 사용자가 원하는 요청을 입력하면, 모델이 # 필요한 함수를 순차적으로 호출할 수 있음 chat_history = [] while True: user_input = input("\nUSER> ") if user_input.lower() == "quit": break ai_response = openai_llm(user_input, chat_history) print(f"A I> {ai_response}")</code>3. 동작 확인예시로, “A 함수를 호출해”라고 입력하면, 콘솔에 함수 호출 과정이 순서대로 찍히고, 최종적으로 “a_func”가 호출된 결과(문자열 "abcdefghijklmnopqrstuvwxyz" 등)가 출력됩니다. 실제로는 모델이 단계마다 z_func를 호출하여 "z" 토큰을 만들고, y_func(z_token="z")를 호출해 "yz"를 만든 뒤, x_func(y_token="yz")를 호출해 "xyz" … 이런 식으로 중간 함수 결과를 축적합니다.콘솔 로그:4. 정리 및 활용 아이디어Function Calling을 통해, 단순 “모델 출력 파싱” 과정을 자동화복잡한 함수 간 의존이 있어도, 모델이 “먼저 필요한 정보를 얻기 위한 함수 → 후속 함수” 순으로 여러 번 호출 가능실제로는 사내 API, DB, 외부 API 등을 호출하여 다양한 작업을 수행할 수 있음함수 개수가 많을수록(10~20개 이상) 모델이 올바른 함수를 찾기 어려울 수 있으므로, 함수명을 직관적으로 지어주고, 시스템 메시지나 함수 description에서 활용 맥락을 자세히 안내해주면 좋습니다.이로써, 서로 의존관계를 가지는 다수의 함수를 OpenAI Function Calling으로 유연하게 구성하는 방법을 살펴봤습니다. ReferenceOpenAI Function Calling 문서OpenAI Cookbook: How to call functions with chat models
이 글에서는 LangGraph 라이브러리의 체크포인트 기능을 이용해, 사용자별로 대화 맥락을 유지할 수 있는 챗봇을 구현해보겠습니다. Anthropic Claude 모델을 활용하여 자연스럽게 대화를 이어가면서, thread_id를 통해 서로 다른 사용자의 대화를 독립적으로 관리하는 방법을 시연합니다.1. 배경: 왜 멀티턴 메모리가 필요한가?지금까지 단순 챗봇을 구성해본 분들은, 한 번의 질의-응답 이후 맥락이 사라지거나, 프로그램이 재시작되면 이전 대화 내용을 잊어버리는 문제를 종종 겪었을 것입니다.예:“내 이름이 홍길동이야” → “반갑습니다, 홍길동님”…(대화 종료 후) 다시 시작하면 홍길동이라는 사실을 잊어버림.LangGraph는 이런 문제를 해결하기 위해 **체크포인트(Checkpoint)**라는 개념을 도입했습니다. 체크포인트는 노드가 실행된 후의 상태(State)를 자동으로 저장해두고, 동일 thread_id로 그래프를 다시 호출하면 그 상태를 자동 로드하여 이어갈 수 있도록 해줍니다.2. 코드 예시다음 코드를 ch4_memory_chatbot.py라 하고, python ch4_memory_chatbot.py로 실행하면 됩니다.<code class="language-plaintext">import os # Anthropic Claude from langchain_anthropic import ChatAnthropic # LangGraph 핵심 from langgraph.graph import StateGraph, START from langgraph.graph.message import add_messages # 체크포인트 from langgraph.checkpoint.memory import MemorySaver # (DB 사용 시 SqliteSaver, PostgresSaver 등 사용 가능) from typing import Annotated from typing_extensions import TypedDict ############################################################################### # 1) 상태(State) 정의 ############################################################################### class State(TypedDict): messages: Annotated[list, add_messages] ############################################################################### # 2) 그래프 빌더 생성 ############################################################################### graph_builder = StateGraph(State) ############################################################################### # 3) LLM ############################################################################### llm = ChatAnthropic( model="claude-3-5-sonnet-20241022", anthropic_api_key=os.environ.get("ANTHROPIC_API_KEY") ) def chatbot_node(state: State): """ state["messages"]를 LLM에게 전달하고, 새 AI 응답을 반환. """ ai_resp = llm.invoke(state["messages"]) return {"messages": [ai_resp]} # 노드 등록 graph_builder.add_node("chatbot", chatbot_node) # START -> chatbot graph_builder.add_edge(START, "chatbot") ############################################################################### # 4) 체크포인터 설정 ############################################################################### memory = MemorySaver() ############################################################################### # 5) 그래프 컴파일 ############################################################################### # compile(...) 시 checkpointer 지정 graph = graph_builder.compile(checkpointer=memory) ################################################################################ # 그래프 시각화를 위한 ASCII 아트 생성 ################################################################################ # - graph.get_graph().draw_ascii()를 호출하여 그래프 구조를 ASCII 아트로 표현 # - 노드와 엣지의 연결 관계를 시각적으로 보여줌 ascii_graph = graph.get_graph().draw_ascii() print(ascii_graph) ############################################################################### # 6) 메인 함수 ############################################################################### def main(): print("==== [ch4] Memory/Checkpoint Demo ====") print("Type 'quit' or 'q' to exit.\n") # 각 유저별 thread_id를 가정 thread_id = input("Enter thread_id (e.g., 'user1'): ").strip() or "default" while True: user_input = input(f"(thread={thread_id}) You: ") if user_input.lower() in ["quit", "q"]: print("Goodbye!") break # graph 실행: # (1) input: {"messages": [("user", user_input)]} # (2) config: {"configurable": {"thread_id": thread_id}} # => LangGraph가 thread_id='...' 체크포인트 상태를 로드 & 업데이트 events = graph.stream( {"messages": [("user", user_input)]}, {"configurable": {"thread_id": thread_id}}, ) # 실행 중 생기는 event를 출력 for event in events: for node_out in event.values(): if "messages" in node_out: last_msg = node_out["messages"][-1] print("Assistant:", last_msg.content) if __name__ == "__main__": main()</code>3. 코드 흐름 분석State(TypedDict) 정의<code class="language-python">class State(TypedDict): messages: Annotated[list, add_messages]</code>챗봇 대화에는 사용자 메시지와 AI(Claude) 메시지를 순서대로 저장하는 messages 리스트가 필요합니다.add_messages를 사용하면 새 메시지를 추가할 때마다 기존 리스트에 “append” 방식으로 쌓입니다.그래프 빌더(StateGraph)graph_builder = StateGraph(State)로 그래프 설계자(Builder)를 생성합니다.노드와 엣지를 등록한 뒤 compile하여 실행 가능한 그래프 객체를 만들어냅니다.LLM 노드(chatbot_node)<code class="language-python">def chatbot_node(state: State): ai_resp = llm.invoke(state["messages"]) return {"messages": [ai_resp]}</code>state["messages"]를 Anthropic Claude에 전달해 새 AI 응답을 생성하는 노드입니다.반환되는 AI 메시지가 messages 리스트에 append되어 대화 내역이 누적됩니다.체크포인터(MemorySaver)<code class="language-python">memory = MemorySaver() graph = graph_builder.compile(checkpointer=memory)</code>이 설정 덕분에 LangGraph가 노드 실행 때마다 상태(State)를 memory에 저장합니다.만약 동일한 thread_id로 다시 그래프를 실행하면, 이전에 저장된 상태를 자동 로드해 여러 턴 대화를 이어갈 수 있습니다.메인 함수thread_id를 입력 받음: 예) user1사용자 메시지를 graph.stream(...)으로 전달할 때,로 지정하면 LangGraph가 해당 스레드의 상태를 로드/업데이트합니다.<code class="language-python">{"configurable": {"thread_id": thread_id}}</code>4. 실행 예시한 터미널에서:<code class="language-plaintext">$ python ch4_memory_chatbot.py +-----------+ | __start__ | +-----------+ * * * +---------+ | chatbot | +---------+ ==== [ch4] Memory/Checkpoint Demo ==== Type 'quit' or 'q' to exit. Enter thread_id (e.g., 'user1'): userA (thread=userA) You: 안녕 Assistant: 안녕하세요! 무엇을 도와드릴까요? (thread=userA) You: 내 이름은 길동이야 Assistant: 길동님, 만나서 반갑습니다. ... </code>또 다른 터미널에서:<code class="language-plaintext">$ python ch4_memory_chatbot.py ==== [ch4] Memory/Checkpoint Demo ==== Type 'quit' or 'q' to exit. Enter thread_id (e.g., 'user1'): userB (thread=userB) You: 안녕, 난 영희야 Assistant: 영희님, 안녕하세요!</code>코드 복사userA와 userB는 서로 다른 thread_id로 대화를 유지하므로, 서로의 대화가 섞이지 않고 독립된 맥락을 이어갑니다.만약 같은 thread_id로 접속하면, LangGraph는 “이전에 저장된 State가 있다”고 보고 과거 메시지를 이어서 참조합니다(프로세스를 종료하기 전까지).5. 확장 아이디어SqliteSaver / PostgresSaverMemorySaver는 파이썬 프로세스를 종료하면 저장된 상태가 사라집니다.SqliteSaver나 PostgresSaver를 쓰면 DB에 저장되므로, 프로그램을 재시작해도 동일 thread_id의 대화를 이어갈 수 있습니다.복수 노드(툴 등) 결합ch2, ch3처럼 툴(검색, DB 조회) 노드를 추가하면, 사용자별로 검색 내역도 messages에 누적되어 “이전에 검색했던 내용”까지 기억하는 챗봇이 됩니다.Human-in-the-loop특정 노드 실행 전/후에 interrupt_before/interrupt_after를 설정해, “사람이 승인해야 다음 단계로 진행”처럼 구현할 수도 있습니다.이때도 체크포인트가 있어야, 중간에 멈췄다가 몇 분/몇 시간 뒤에 다시 재개 가능합니다.마무리이상으로, LangGraph의 체크포인트 기능을 활용해 여러 턴 대화(멀티턴)와 사용자별(thread_id 기반) 대화 맥락 유지를 구현하는 방법을 살펴봤습니다.핵심 포인트:State에 messages 리스트를 넣어두고,compile(checkpointer=...)로 그래프 실행 결과를 저장,graph.stream(..., {"configurable": {"thread_id": "..."}})로 이전 기록을 로드.이를 통해 간단히 사용자별 맞춤형 기억을 유지할 수 있으며, 좀 더 고도화하면 DB 연동으로 서버 재시작에도 대화 이력이 남도록 만들 수 있습니다.
ch1에서는 LangGraph를 사용한 기본 챗봇을, ch2에서는 특정 상황에서 도구(검색 엔진)를 자동 호출하는 흐름을 살펴봤습니다. 하지만, ch2에서 우리는 LangGraph가 미리 만들어놓은 ToolNode & tools_condition 을 그대로 썼죠. 이번 ch3에서는 이러한 편의 기능을 직접 구현해봄으로써, LangGraph의 노드 정의와 조건부 라우팅(conditional_edges)을 보다 깊이 이해해보겠습니다.1. 준비 사항가상환경 & 패키지 설치<code class="language-plaintext">python -m venv .venv source .venv/bin/activate pip install -U langgraph langsmith langchain_anthropic tavily-python langchain_community</code>API Key 설정Anthropic: export ANTHROPIC_API_KEY="..."Tavily: export TAVILY_API_KEY="..."기본 개념LangGraph에서 노드(Node) 는 파이썬 함수(또는 객체의 __call__)로 구현되며,“START -> (여러 노드) -> END” 형태의 흐름을 edges로 연결한다.conditional_edges를 통해 조건부 분기를 제어할 수 있다.3. 전체 코드아래 예시는 Anthropic Claude와 TavilySearchResults를 연동한 LangGraph 챗봇으로, LLM이 “검색이 필요하다”고 판단할 때, 직접 만든 BasicToolNode가 실행되어 웹 검색을 수행합니다.<code class="language-python">import os import json # Anthropic Claude from langchain_anthropic import ChatAnthropic # LangGraph from langgraph.graph import StateGraph, START, END from langgraph.graph.message import add_messages # Tavily 검색 툴 (웹 검색) from langchain_community.tools.tavily_search import TavilySearchResults # typing from typing import Annotated from typing_extensions import TypedDict # langchain-core에서 ToolMessage (툴 호출 결과를 담아 LLM에 전달하기 위함) from langchain_core.messages import ToolMessage ############################################################################### # 1) 상태(State) 정의 ############################################################################### class State(TypedDict): # messages: 사용자/AI 메시지를 누적 저장 messages: Annotated[list, add_messages] ############################################################################### # 2) 그래프 빌더 생성 ############################################################################### graph_builder = StateGraph(State) ############################################################################### # 3) LLM 및 툴 초기화 ############################################################################### # - TavilySearchResults: 검색어를 넣으면 JSON 형식으로 결과 반환 search_tool = TavilySearchResults( max_results=2, tavily_api_key=os.environ.get("TAVILY_API_KEY") # 환경변수에서 가져오기 ) # - Anthropic Claude llm = ChatAnthropic( model="claude-3-5-sonnet-20240620", anthropic_api_key=os.environ.get("ANTHROPIC_API_KEY") ) # - LLM에 툴 목록을 "bind"하여, # LLM이 tool_calls(JSON) 형태로 특정 툴을 호출할 수 있음 llm_with_tools = llm.bind_tools([search_tool]) ############################################################################### # 4) 노드: chatbot ############################################################################### def chatbot_node(state: State): """ 1) state["messages"]를 LLM에게 전달 2) LLM이 필요 시 tool_calls를 생성 (search_tool 등) 3) 새 메시지를 반환 -> LangGraph가 append """ ai_resp = llm_with_tools.invoke(state["messages"]) return {"messages": [ai_resp]} ############################################################################### # 5) 노드: BasicToolNode (직접 구현) ############################################################################### # - LangGraph가 기본 제공하는 ToolNode가 아니라, # LLM이 호출하겠다고 선언한 tool_calls를 직접 처리하는 로직 class BasicToolNode: """ LLM의 마지막 메시지에 tool_calls가 있으면, 그에 따라 실제 툴을 실행하고 ToolMessage를 생성한다. """ def __init__(self, tools: list): # tools_by_name = {툴_이름: 툴_인스턴스} self.tools_by_name = {t.name: t for t in tools} def __call__(self, inputs: dict): # inputs에는 "messages" 리스트가 들어있음 if messages := inputs.get("messages", []): last_msg = messages[-1] # 가장 최근 AI 메시지 else: raise ValueError("No messages found in input.") outputs = [] # AI 메시지에 tool_calls가 있는지 확인 if hasattr(last_msg, "tool_calls"): for call_info in last_msg.tool_calls: tool_name = call_info["name"] args = call_info["args"] # 툴 이름에 맞춰 실제 invoke if tool_name in self.tools_by_name: result = self.tools_by_name[tool_name].invoke(args) # 검색 결과(등등)를 ToolMessage 형태로 담아 반환 # -> chatbot 노드에서 이어받아 최종 답변에 활용 outputs.append( ToolMessage( content=json.dumps(result), name=tool_name, tool_call_id=call_info["id"], ) ) return {"messages": outputs} ############################################################################### # 6) 노드 등록 ############################################################################### graph_builder.add_node("chatbot", chatbot_node) tool_node = BasicToolNode([search_tool]) graph_builder.add_node("tools", tool_node) ############################################################################### # 7) 조건부 라우팅 ############################################################################### # - chatbot 노드가 실행된 후, LLM이 도구를 호출했는지 여부를 판단해 # "tools" 노드로 갈지, "END"로 갈지 결정 def route_tools(state: State): """ chatbot 노드가 끝난 직후 실행되는 함수 - state["messages"][-1] 에 tool_calls가 있으면 'tools' - 없으면 'END' """ if messages := state.get("messages", []): last_msg = messages[-1] # tool_calls가 있고, 갯수가 > 0이면 'tools' if hasattr(last_msg, "tool_calls") and last_msg.tool_calls: return "tools" # 그렇지 않으면 'END' return END # - add_conditional_edges("chatbot", route_tools, {"tools":"tools", END:END}) graph_builder.add_conditional_edges( "chatbot", route_tools, { "tools": "tools", END: END } ) # - tools -> chatbot: 툴 실행 후 다시 chatbot 노드로 돌아가서 최종 답변을 완성 graph_builder.add_edge("tools", "chatbot") # - START -> chatbot graph_builder.add_edge(START, "chatbot") ############################################################################### # 8) 그래프 컴파일 ############################################################################### graph = graph_builder.compile() ################################################################################ # 그래프 시각화를 위한 ASCII 아트 생성 ################################################################################ # - graph.get_graph().draw_ascii()를 호출하여 그래프 구조를 ASCII 아트로 표현 # - 노드와 엣지의 연결 관계를 시각적으로 보여줌 ascii_graph = graph.get_graph().draw_ascii() print(ascii_graph) ############################################################################### # 9) 메인 함수 ############################################################################### def main(): print("==== [ch3] Custom BasicToolNode Demo ====") print("Type 'quit' or 'q' to exit.\n") while True: user_input = input("User: ") if user_input.lower() in ["quit", "q", "exit"]: print("Goodbye!") break # 그래프를 단계별로 실행 # "messages": [("user", user_input)] => 대화 목록에 새 사용자 메시지 추가 events = graph.stream({"messages": [("user", user_input)]}) # 실행 중 발생하는 모든 노드의 업데이트를 받아 메시지가 생성되면 출력 for event in events: for node_output in event.values(): if "messages" in node_output: last_msg = node_output["messages"][-1] # AIMessage 혹은 ToolMessage print("Assistant:", last_msg.content) if __name__ == "__main__": main()</code>4. 코드 해설chatbot_nodestate["messages"]를 LLM(Claude)에 전달해 새 응답을 생성.Claude가 “검색이 필요하다”고 판단하면 응답에 tool_calls를 담아 돌려준다.BasicToolNode (직접 구현)LangGraph의 빌트인 ToolNode와 유사한 역할을 하지만, 커스텀 로직이 필요할 경우 이처럼 직접 짤 수 있다.마지막 AI 메시지(messages[-1])에 tool_calls가 있으면, 해당 툴을 invoke(args)로 실행.그 결과를 ToolMessage로 만들어 대화에 추가.한 번 검색을 수행하면, 다음 턴에 다시 chatbot_node로 돌아와 최종 답변이 완성되는 구조.조건부 라우팅 (route_tools)chatbot 노드가 끝난 뒤 실행되는 함수로,메시지에 tool_calls가 있으면 "tools", 없으면 "END"로 분기.이후 "tools" -> "chatbot"으로 돌아와 LLM이 새로운 정보를 활용할 수 있게 된다.메인 함수사용자 입력 -> graph.stream(...) -> 순차적으로 노드가 실행되며 메시지를 추가 -> 결과 출력사용자가 “quit”를 입력하기 전까지 반복.5. 실행 예시<code class="language-python">$ python ch3_chatbot_with_tools_using_customnode.py +-----------+ | __start__ | +-----------+ * * * +---------+ | chatbot | +---------+ . . .. .. . . +-------+ +---------+ | tools | | __end__ | +-------+ +---------+ ==== [ch3] Custom BasicToolNode Demo ==== Type 'quit' or 'q' to exit. User: 안녕 Claude Assistant: 안녕하세요! 어떻게 도와드릴까요? User: LangGraph가 뭐야? Assistant: [{'text': "Let me look that up for you...", 'type': 'text'}, {'id': 'toolu_01A...', 'input': {'query': 'LangGraph'}, ...}] Assistant: [{"url": "https://github.com/langchain-ai/langgraph", "content": "LangGraph is a library for ..."}] Assistant: LangGraph는 ....</code>Claude가 “검색해야겠다”고 판단하면, tool_calls JSON이 AI 메시지에 들어 있음 → BasicToolNode가 search_tool을 실제로 호출 → 그 결과를 ToolMessage로 만들어 대화에 추가 → 다시 chatbot_node에서 최종 요약/답변을 준다.6. add_conditional_edges 좀 더 살펴보기노드(Node)가 완료된 뒤, 현재 상태(State)를 분석하여 다음에 어디로 갈지 분기(조건부 라우팅) 하고 싶을 때 사용합니다. 즉, “조건에 따라 서로 다른 노드로 이동”하는 흐름을 간단히 정의할 수 있습니다.일반적인 add_edge("A", "B")는 “A 후에 무조건 B”로 연결되지만,add_conditional_edges("A", condition_fn, {...})는 “A 후에 condition_fn의 결과값에 따라 여러 갈래”로 분기할 수 있습니다.함수 문법<code class="language-python">StateGraph.add_conditional_edges( from_node: str, condition: Callable[[Any], str | list[str]], mapping: dict[str, str] = {}, ... ) -> None</code>from_node: str어떤 노드가 끝난 뒤 이 조건부 로직을 적용할지 지정.예: "chatbot" → chatbot 노드가 실행을 마치면 condition 함수를 호출하여 분기 결정.condition: Callable[[Any], str | list[str]]실제 분기 로직이 들어 있는 함수(“조건 함수”).그래프의 현재 State(예: TypedDict)를 입력으로 받아, 다음으로 이동할 노드 이름(문자열)을 반환.하나의 문자열을 반환하면 “단일 분기”, 문자열 리스트를 반환하면 “여러 노드로 병렬 분기”도 가능.예시:<code class="language-python">def route_tools(state: State) -> str: last_msg = state["messages"][-1] if hasattr(last_msg, "tool_calls") and last_msg.tool_calls: return "tools" return "END"</code>mapping: dict[str, str]condition 함수가 반환한 문자열(또는 문자열 중 하나)을 실제 노드 이름 혹은 특수 키(END)에 매핑.예: { "tools": "tools", "end": END }condition이 "tools"를 반환하면 실제로 tools 노드로 이동."end"를 반환하면 그래프 종료(END).만약 condition이 "my_tools" 같은 임의 문자열을 반환하고, mapping에 {"my_tools": "tools"} 라고 작성하면, 실제로는 "tools" 노드로 이동시키는 식으로 커스터마이징 가능.보통은 "tools" → "tools", "END" → END 처럼 간단하게 1:1 매핑하는 경우가 많습니다.리턴(반환) 값add_conditional_edges 자체는 그래프에 조건부 엣지를 추가하고 None을 반환합니다.이후 그래프가 from_node를 마친 시점에 내부적으로 condition(state)를 호출해, 반환값을 mapping으로 해석하여 다음 노드를 결정하게 됩니다.7. 마무리 및 확장직접 구현한 ToolNode를 통해 LangGraph에서 다양한 모듈(예: 검색, DB 조회, 사내 API 호출 등)을 자유롭게 연결할 수 있습니다.함수형으로 만들 수도 있고, 이번처럼 클래스(__call__)로 만들어 노드 로직을 더 체계적으로 관리할 수도 있습니다.조건부 라우팅 역시 직접 route_tools 함수를 짜보면, LangGraph가 제공하는 편의 함수(tools_condition)의 내부 동작을 파악하는 데 도움이 됩니다.이상으로, ch3: LangGraph에서 직접 만든 ToolNode로 검색 툴을 연동해보는 방법을 소개해드렸습니다!
우리는 ch1에서 LangGraph의 StateGraph를 만들고, 간단한 노드(chatbot)와 엣지(START->chatbot->END)를 연결해, “사용자 입력 -> LLM 응답”의 기초적인 챗봇을 완성했습니다. 그 결과, Claude가 이미 알고 있는 지식 범위 내에서는 문제없이 대화를 이어갈 수 있었죠. 하지만 LLM이 미처 학습하지 못했거나 최신 정보를 요구하는 상황에서는 응답이 부정확해질 수 있었습니다. 이를 해결하기 위해 검색 엔진 등의 외부 도구(tool) 를 연결해 필요한 데이터를 실시간으로 가져오는 방식을 고려해볼 수 있습니다. 이번 ch2에서는 LangGraph에 툴 노드(ToolNode) 를 추가해, LLM이 필요시 스스로 검색 툴을 사용할 수 있도록 만들고, 조건부 분기(conditional edges)를 적용해 LLM이 검색을 원하는 경우와 원치 않는 경우를 구분하여 처리합니다. 이를 통해, 사용자 질문에 대해 LLM이 필요 시 웹 검색을 수행하고, 그 결과를 바탕으로 대답을 보강할 수 있게 됩니다.1. 사전 준비 사항가상환경 활성화<code class="language-plaintext">python -m venv .venv source .venv/bin/activate</code>필요 라이브러리 설치<code class="language-plaintext">pip install -U langgraph langsmith langchain_anthropic tavily-python langchain_community</code>환경변수 설정Anthropic API 키: export ANTHROPIC_API_KEY="..."Tavily API 키: export TAVILY_API_KEY="..."(Windows PowerShell: $env:ANTHROPIC_API_KEY="..." 식)2. 코드 전체 (ch2_chatbot_with_tools.py)아래 코드를 chatbot_with_tools.py로 저장한 뒤, python chatbot_with_tools.py로 실행해보세요.<code class="language-plaintext">import os # Anthropic Claude 연결 from langchain_anthropic import ChatAnthropic # LangGraph 핵심 구성요소 # - StateGraph: 그래프 정의 # - START, END: 시작/종료 지점 # - add_messages: 새로운 메시지를 State에 append하는 편의함수 from langgraph.graph import StateGraph, START, END from langgraph.graph.message import add_messages # 웹 검색 툴 (Tavily) from langchain_community.tools.tavily_search import TavilySearchResults # 미리 만들어진 'ToolNode'와 'tools_condition' (LangGraph 제공) # - ToolNode: 여러 툴(도구) 실행을 자동 처리 # - tools_condition: "LLM이 툴을 호출했는지" 보고 다음 노드를 결정 from langgraph.prebuilt import ToolNode, tools_condition # 타입 정의 from typing import Annotated from typing_extensions import TypedDict ################################################################################ # 1) State(상태) 정의 ################################################################################ # - messages: 사용자/AI 메시지를 저장해두는 리스트 # - add_messages: 새 메시지를 append class State(TypedDict): messages: Annotated[list, add_messages] ################################################################################ # 2) 그래프 빌더 생성 ################################################################################ # - 우리가 만든 State 구조를 기반으로 동작하는 StateGraph # - 노드와 엣지를 등록한 후, compile() 하면 실행 가능해짐 graph_builder = StateGraph(State) ################################################################################ # 3) LLM + 툴 생성 ################################################################################ # (A) Tavily 웹 검색 툴 search_tool = TavilySearchResults( max_results=2, # 검색 결과 최대 개수 tavily_api_key=os.environ.get("TAVILY_API_KEY") # 환경변수에서 가져오기 ) # (B) Anthropic Claude llm = ChatAnthropic( model="claude-3-5-sonnet-20241022", # 원하시는 모델 버전으로 수정 가능 anthropic_api_key=os.environ.get("ANTHROPIC_API_KEY") ) # (C) LLM + 툴 바인딩 # - LLM이 JSON 포맷을 통해 툴을 "호출"할 수 있게 설정 llm_with_tools = llm.bind_tools([search_tool]) ################################################################################ # 4) 노드(Node) 정의 ################################################################################ # (1) chatbot 노드 # - state["messages"]를 보고 LLM이 답변 # - 필요하면 (tool_calls)를 포함하여, 툴을 호출하도록 지시 def chatbot_node(state: State): ai_response = llm_with_tools.invoke(state["messages"]) return { "messages": [ai_response] } # (2) tools 노드 # - LLM 메시지에 툴 호출 정보(tool_calls)가 있으면 # 실제 검색 툴을 실행하고, 그 결과를 messages에 append # -> LangGraph에는 이미 'ToolNode'라는 편의 클래스가 있으므로 직접 구현 필요 없음 # 이번에는 prebuilt된 ToolNode를 사용해보겠습니다. tool_node = ToolNode(tools=[search_tool]) ################################################################################ # 5) 그래프 빌더에 노드 등록 ################################################################################ # - "chatbot" 노드 -> chatbot_node(함수) graph_builder.add_node("chatbot", chatbot_node) # - "tools" 노드 -> tool_node(객체) # => tool_node(...) 실행 시 LLM이 요청한 툴들을 병렬/직렬 등으로 실행 가능 graph_builder.add_node("tools", tool_node) ################################################################################ # 6) 조건부 엣지(conditional_edges) 설정 ################################################################################ # - LangGraph의 핵심: "조건부 엣지"로 다음 노드를 동적으로 결정 # - tools_condition: # => "LLM이 툴을 호출했으면 tools 노드로, 아니면 END로" 라우팅 # - chatbot 노드가 실행된 후, tools_condition로 분기 # (예: "search_tool" 호출 요청이 있으면 'tools'로 이동 / 없으면 'END') graph_builder.add_conditional_edges( "chatbot", tools_condition, # condition 함수 { "tools": "tools", # condition이 "tools"라 반환하면, 실제로 "tools" 노드로 이동 END: END # condition이 END라 반환하면 그래프 종료 } ) ################################################################################ # 7) 추가 엣지 설정 ################################################################################ # - "tools" -> "chatbot" : 툴 실행 후 다시 LLM에 돌아와 응답을 마무리 # - START -> "chatbot" : 시작하면 곧바로 챗봇 노드부터 실행 graph_builder.add_edge("tools", "chatbot") graph_builder.add_edge(START, "chatbot") ################################################################################ # 8) 컴파일 ################################################################################ # - 이렇게 해서 완성된 노드/엣지 구조를 실제로 "실행할 수 있는" 그래프로 변환 graph = graph_builder.compile() ################################################################################ # 그래프 시각화를 위한 ASCII 아트 생성 ################################################################################ # - graph.get_graph().draw_ascii()를 호출하여 그래프 구조를 ASCII 아트로 표현 # - 노드와 엣지의 연결 관계를 시각적으로 보여줌 ascii_graph = graph.get_graph().draw_ascii() print(ascii_graph) ################################################################################ # 9) 메인 함수 ################################################################################ def main(): print("==== [ch2] LangGraph + Tools (Conditional) Demo ====") print("Type 'quit' or 'q' to exit.\n") while True: user_input = input("User: ") if user_input.lower() in ["quit", "q", "exit"]: print("Goodbye!") break # 그래프 실행 # - 입력 형태: {"messages": [("user", user_input)]} # - chatbot 노드 -> tools_condition -> (tools or end) -> ... # - 툴 실행이 발생하면 "tools" 노드가 검색 수행, 결과를 messages에 저장 # - 이후 다시 chatbot 노드에서 최종 답변 events = graph.stream({"messages": [("user", user_input)]}) # 실행 중 발생하는 event(상태 업데이트)마다 새 메시지가 있다면 출력 for event in events: for node_output in event.values(): if "messages" in node_output: # 가장 마지막 메시지(새로운 답변) last_msg = node_output["messages"][-1] # AIMessage의 .content 속성 출력 print("Assistant:", last_msg.content) if __name__ == "__main__": main()</code>3. 주요 흐름 설명chatbot 노드가 사용자 메시지를 보고 Claude에게 질의Claude가 “외부 검색이 필요하다”고 판단하면, tool_calls에 search_tool을 호출하는 JSON을 담아 반환조건부 엣지 (tools_condition)LLM 응답에 툴 호출이 있으면 "tools" 노드로 이동, 없으면 END로 종료tools 노드실제 TavilySearchResults를 호출해 검색 결과(JSON)를 messages에 추가이후 다시 "chatbot" 노드로 돌아가, 검색 결과를 이용해 최종 답변 생성메인 루프사용자 입력 -> 그래프 stream -> (필요 시 검색) -> 최종 답변 출력“quit” 입력 시 종료4. 실행 예시 <code class="language-plaintext">$ python ch2_chatbot_with_tools.py +-----------+ | __start__ | +-----------+ * * * +---------+ | chatbot | +---------+ . . .. .. . . +-------+ +---------+ | tools | | __end__ | +-------+ +---------+ ==== [ch2] LangGraph + Tools (Conditional) Demo ==== Type 'quit' or 'q' to exit. User: LangGraph는 뭐야? Assistant: (Claude가 답변 중...) Assistant: ... (검색 필요 판단) tool_calls -> search_tool Assistant: ... (검색 결과) ... Assistant: LangGraph는 ~~~ 입니다!</code>Claude가 학습된 지식만으로 답할 수 있으면 툴을 안 쓰고 바로 끝(END)추가 정보가 필요하면 tool_calls를 통해 search_tool을 실행하고, 검색 결과를 메시지로 받아와 더 풍부한 답변을 구성합니다.이상으로, LangGraph + Anthropic Claude + TavilySearchResults를 이용한 도구(검색) 활용 챗봇 예시(ch2)를 살펴봤습니다. 소스코드는 간단하지만 add_conditional_edges에 대해 생소하시다면 완벽히 이해가 되지 않을 수 있습니다. 이 부분에는 다음 챕터에서 살펴보겠습니다.